How AI Automation is Transforming Data Analysis: A Comprehensive Guide
- pengarhehe
- Mar 12
- 6 min read

AI Automation Data Analysis
In an era where data is the lifeblood of decision-making, AI automation is revolutionizing how we process, analyze, and interpret information. Imagine a world where tedious data tasks—collecting figures from countless sources, scrubbing errors, or generating reports—are handled seamlessly by intelligent systems, leaving humans free to focus on strategy and innovation. That world is here, and it’s powered by AI automation in data analysis.
This comprehensive guide dives deep into how AI automation is transforming data analysis, exploring its mechanics, benefits, challenges, real-world applications, best practices, and what’s on the horizon for 2025. Whether you’re a business owner aiming to streamline operations, a data scientist curious about the latest tools, or simply an enthusiast eager to understand this technological shift, this article is your one-stop resource. By the end, you’ll not only grasp why AI automation is a game-changer but also how to leverage it effectively.
Understanding AI Automation in Data Analysis
What is AI Automation in Data Analysis?
At its core, AI automation in data analysis is the application of artificial intelligence technologies to streamline and enhance the data analysis process. It’s about letting machines handle the heavy lifting—tasks like gathering data, cleaning it up, crunching numbers, and even presenting insights in a digestible format. Think of it as a super-smart assistant that doesn’t tire, doesn’t err (when trained right), and works at lightning speed.
AI automation leverages tools like machine learning (ML), natural language processing (NLP), and robotic process automation (RPA) to take over repetitive or complex tasks that once demanded hours of human effort. The result? Faster insights, fewer mistakes, and a sharper focus on what the data actually means.
Types of AI Powering Data Analysis
AI isn’t a monolith—it comes in flavors, each serving a unique role in data analysis:
Machine Learning (ML): This is the brain behind predictive analytics. ML algorithms learn from historical data to forecast trends, spot anomalies, or classify information. For example, an ML model might predict customer churn based on past behavior.
Natural Language Processing (NLP): Ever wondered how AI makes sense of customer reviews or social media chatter? NLP translates unstructured text into actionable insights, perfect for sentiment analysis or chatbot-driven data collection.
Robotic Process Automation (RPA): RPA is the workhorse for mundane tasks—think data entry, report generation, or pulling stats from multiple systems. It’s less about thinking and more about doing.
Stages of Data Analysis AI Can Automate
Data analysis isn’t a single step—it’s a pipeline, and AI can optimize every stage:
Data Collection: AI tools can scrape websites, pull from APIs, or sync with databases in real time, ensuring you’ve got the latest info without lifting a finger.
Data Cleaning: Messy data? AI identifies duplicates, fills missing values, and standardizes formats faster than any human could.
Data Analysis: Here’s where the magic happens—AI crunches numbers, uncovers patterns, and delivers insights, often in seconds.
Data Visualization: Need a chart or dashboard? AI-powered tools like Tableau or Power BI can auto-generate visuals that make complex data crystal clear.
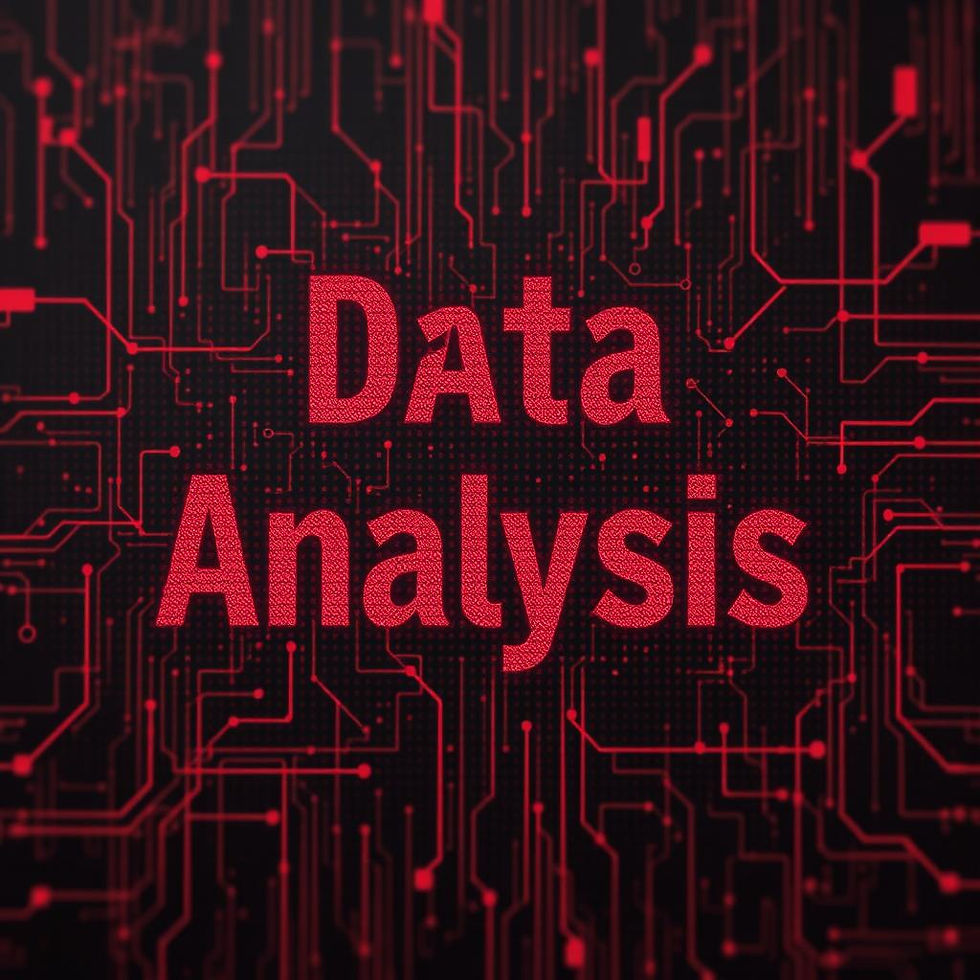
Benefits of AI Automation in Data Analysis
Why bother with AI automation? The perks are transformative, reshaping how businesses operate. Here’s a breakdown:
1. Increased Efficiency
AI doesn’t sleep, doesn’t take coffee breaks, and doesn’t get bogged down by massive datasets. Tasks that once took days—like analyzing sales trends across regions—can now be done in minutes. This speed translates to quicker decisions and a competitive edge.
2. Improved Accuracy
Humans make mistakes. We miss decimals, misread rows, or overlook outliers. AI, when properly trained, doesn’t. It delivers consistent, precise results, making it invaluable for high-stakes fields like finance or healthcare.
3. Scalability
Got a dataset that’s growing by the terabyte? No problem. AI scales effortlessly, handling everything from small spreadsheets to big data lakes without breaking a sweat.
4. Cost Savings
Automation cuts labor costs by reducing the need for manual data grunt work. Plus, AI can spot inefficiencies—like overstocked inventory—saving money in the long run. Tools like Activecampaign even integrate AI with CRM to optimize workflows further.
5. Enhanced Decision-Making
AI doesn’t just process data—it reveals the “why” behind the numbers. By identifying hidden trends or correlations, it empowers leaders to make smarter, evidence-based choices.
Table: Benefits of AI Automation in Data Analysis
Benefit | Description | Example Use Case |
Increased Efficiency | Speeds up data processing | Real-time inventory tracking |
Improved Accuracy | Minimizes errors | Medical diagnosis validation |
Scalability | Manages growing data volumes | Social media sentiment analysis |
Cost Savings | Reduces labor and operational costs | Automated financial reporting |
Enhanced Decision-Making | Uncovers actionable insights | Marketing campaign optimization |
Challenges and Limitations of AI Automation in Data Analysis
AI isn’t a silver bullet—it comes with hurdles that need addressing. Here’s what to watch out for:
1. Data Privacy Concerns
AI thrives on data, but feeding it sensitive info—like customer details or health records—raises red flags. Compliance with laws like GDPR or HIPAA is non-negotiable, and breaches can be costly.
2. Algorithmic Bias
Garbage in, garbage out. If the data training an AI model is skewed (say, favoring one demographic), the output will be too. This can lead to flawed insights or even ethical dilemmas.
3. Skill Gaps
AI isn’t plug-and-play. You need data scientists to build models, IT pros to maintain systems, and analysts to interpret results. Finding this talent can be tough, especially for smaller firms.
4. High Initial Costs
Setting up AI—think software licenses, hardware upgrades, and training—requires upfront investment. While the ROI is strong, the entry barrier can deter some businesses.
5. Over-Reliance Risks
AI is a tool, not a boss. Leaning too heavily on automation without human oversight can lead to blind spots—like misinterpreting context or missing the big picture.
Real-World Examples of AI Automation in Data Analysis
Seeing AI in action makes its impact tangible. Here are some standout cases:
1. Retail: Customer Behavior Analysis
Retail giants use AI to dissect shopping habits. By analyzing purchase histories and online clicks, AI predicts what customers want next, powering personalized ads. It’s why your favorite store seems to know you so well.
2. Healthcare: Predictive Diagnostics
Hospitals deploy AI to scan patient data—think X-rays or blood tests—to flag early signs of illness. A model might spot lung cancer patterns months before symptoms, saving lives through early intervention.
3. Finance: Fraud Detection
Banks use AI to monitor transactions in real time. If a $5,000 charge pops up in a new country, AI flags it as suspicious, protecting accounts faster than any human team could.
4. Manufacturing: Predictive Maintenance
Factories embed sensors in machines, and AI analyzes the data to predict breakdowns. Fixing a motor before it fails cuts downtime and repair bills—a win for efficiency.
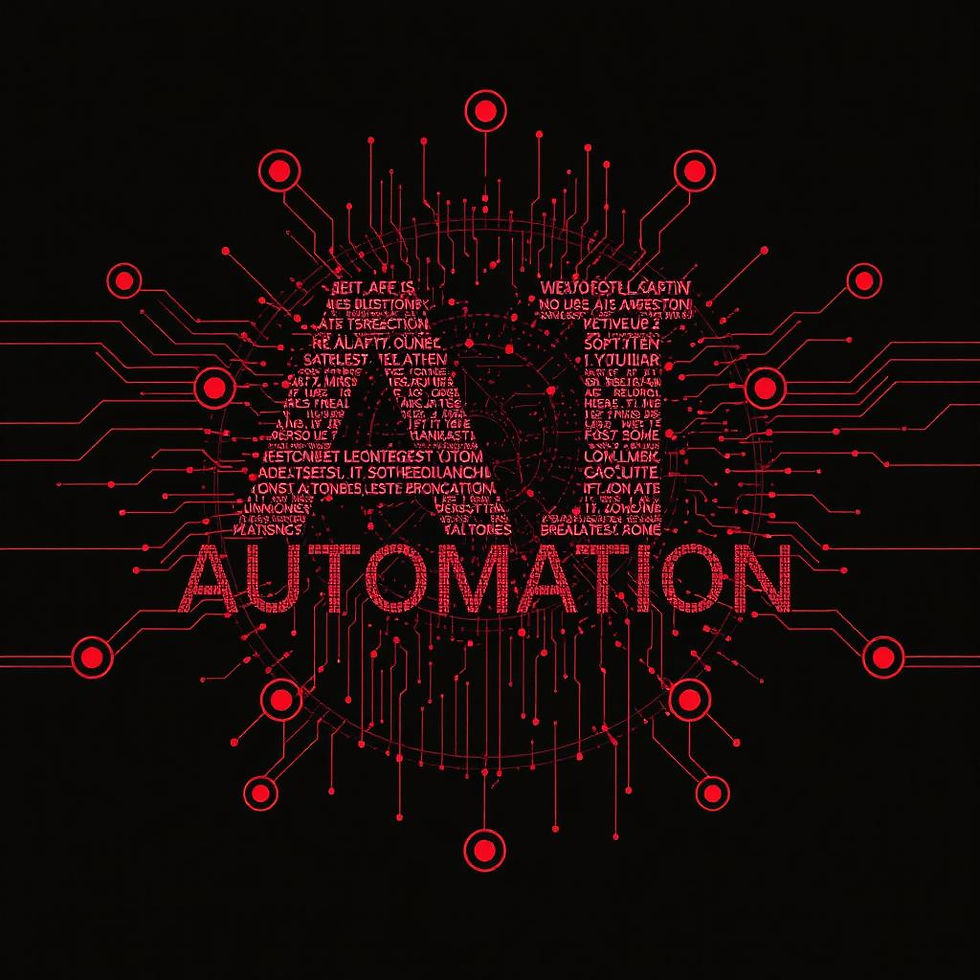
Best Practices for Implementing AI Automation in Data Analysis
Ready to jump in? Here’s how to do it right:
1. Define Clear Goals
What’s your endgame? Faster reports? Better forecasts? Nail down your objectives to steer the AI strategy.
2. Pick the Right Tools
Match tools to tasks. For content-driven data analysis, Copyspace.ai can streamline insights, while Activecampaign excels in customer data automation. Explore our AI Automation Tools guide for more options.
3. Prioritize Data Quality
AI is only as good as its fuel. Clean, accurate, and diverse data ensures reliable results.
4. Train and Monitor Models
AI isn’t set-it-and-forget-it. Regular updates and performance checks keep it sharp and relevant.
5. Blend AI with Human Insight
Let AI crunch the numbers, but keep humans in the loop to interpret nuances and make final calls.
Future Trends in AI Automation for Data Analysis (2025)
What’s next? Here’s what 2025 holds:
1. AI Hardware Boosts
Faster chips and quantum computing will turbocharge AI, slashing analysis times even further.
2. Ethical AI Focus
Expect stricter guidelines to curb bias and ensure transparency, making AI a trusted partner.
3. Tech Synergies
AI will team up with IoT for real-time data feeds and blockchain for secure analysis, creating powerful ecosystems.
4. Agentic AI Rise
Autonomous AI agents will handle entire workflows—like running a full sales analysis—needing just a nudge to start.
FAQ: AI Automation in Data Analysis
What is AI automation in data analysis?
It’s using AI to automate data tasks—collecting, cleaning, analyzing, and visualizing—making the process faster and smarter.
How does AI improve data accuracy?
By cutting human error and spotting patterns with precision, AI ensures cleaner, more reliable insights.
What challenges does AI automation face?
Privacy risks, biased models, skill shortages, setup costs, and over-reliance are the big ones.
How can businesses get started?
Set goals, pick tools, ensure data quality, train models, and pair AI with human expertise.
Conclusion
AI automation is rewriting the rules of data analysis, turning a once-laborious process into a swift, precise, and scalable powerhouse. Yes, there are hurdles—privacy, costs, skills—but the rewards are undeniable: efficiency, accuracy, and insights that drive success. As we head into 2025, staying ahead means embracing these tools, refining strategies, and keeping humans in the driver’s seat.
Want more? Dive into our posts on AI Software or AI in Customer Service for deeper dives.
Comments